Regression analysis is a vitally important statistical tool, with major advancements made by both practical data analysts and statistical theorists. In this book, Raymond H. Myers provides a solid foundation in classical regression, while introducing modern techniques. Throughout the book, a broad spectrum of applications are included from the physical sciences, engineerin Regression analysis is a vitally important statistical tool, with major advancements made by both practical data analysts and statistical theorists. In this book, Raymond H. Myers provides a solid foundation in classical regression, while introducing modern techniques.
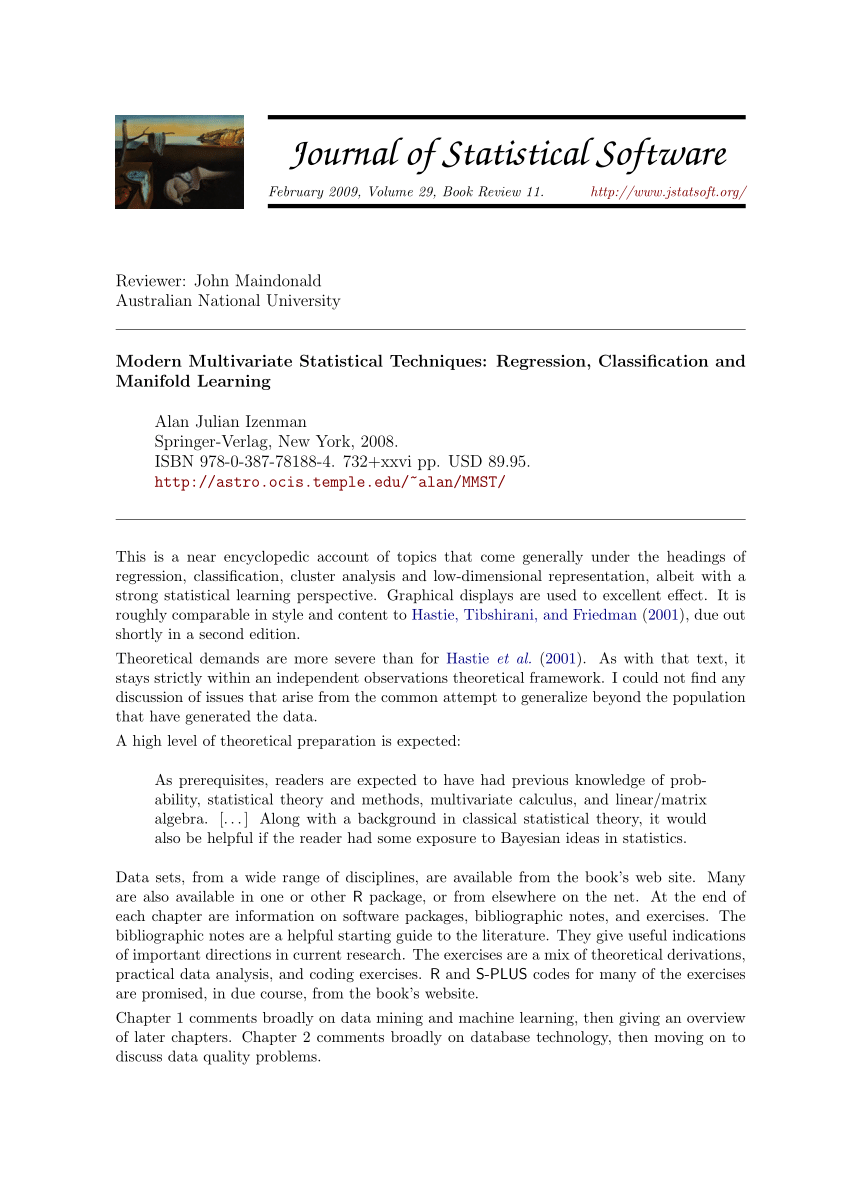
Throughout the book, a broad spectrum of applications are included from the physical sciences, engineering, biology, management, and economics.
Classical And Modern Regression With Applications Pdf Download
,1Population and Social Statistics, Kenya National Bureau of Statistics, Nairobi, Kenya2Department of Statistics and Actuarial Sciences, Jomo Kenyatta University of Agriculture and Technology, Nairobi, Kenya. 2018, 186-200 DOI: 10.12691/ajams-6-5-3 Copyright © 2018 Science and Education Publishing Cite this paper: Pius Miri Ng’ang’a, Antony Waititu Gichuhi, Antony Wanjoya, Thomas Mageto. Modelling Diabetes Mellitus among Adult Kenyan Population Using Artificial Neural Network.
American Journal of Applied Mathematics and Statistics. 2018; 6(5):186-200. Doi: 10.12691/ajams-6-5-3. Correspondence to: Pius Miri Ng’ang’a, Population and Social Statistics, Kenya National Bureau of Statistics, Nairobi, Kenya.
Artificial Neural Network (ANN) is a parallel connection of a set of nodes called neurons which mimic biological neural system. Statistically, ANN represents a class of non-parametric models which is capable of approximating a non-linear function by a composition of low dimensional ridge functions. This study aimed at modeling diabetes mellitus among adult Kenyan population using 2015 stepwise survey data from Kenya National Bureau of Statistics.
Data analysis was carried out using R statistical software version 3.5.0. Among the input variables Age, Sex, Alcoholic status, Sugar consumption, Physical Inactivity, Obesity status, Systolic and Diastolic blood pressure had a significant relationship with diabetic status at 5% level of significance.
A multi layered feed-forward neural network with a back propagation algorithm and a logistic activation function was used. Considering a parsimonious model, the model selected had the eight input variables with two neurons in the hidden layer since it gave a minimum MSE of 0.0580 reported. 75% of data was used for training while 25% was used for testing. The sensitivity of the trained network was reported as 75% while specificity was 94.29%. The overall accuracy of the model was 84.64%.
This implied that the model could correctly classify an individual as either diabetic or not with an accuracy rate of 84.64%. A 10-fold cross validation was carried out and an average MSE of 0.0686 reported.
Kolmogorov-Smirnov test of normality was carried out and at 5% level of significance, for most parameter estimates, we failed to reject the null hypothesis and concluded that the network parameter estimates were asymptotically normal and consistent. With a good choice of risk factors for diabetes, neural network structures could be successfully used to accurately model diabetes melitus among Kenyan adult population.